Don't Drop That Outlier! It Might be Important
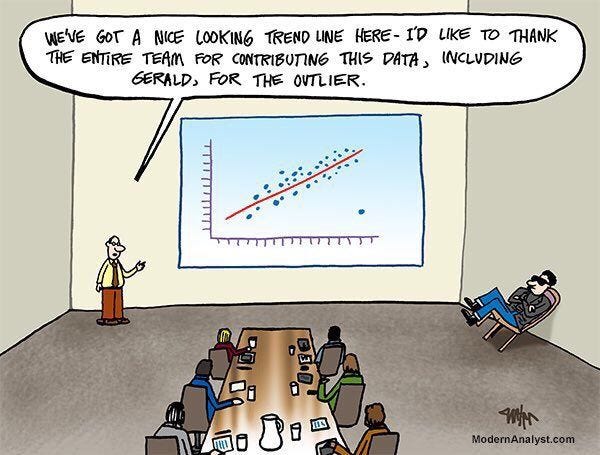
We know that outliers are data points that are extreme or significantly different from the others.
However, it would be best not to drop the outlier without further analysis.
Outliers might affect the data statistic, but If you only and only care about statistical results, then removing the outlier could be an option.
If that’s the reason, then you should ask these questions before deciding to drop them:
Is the outlier because of error measurement or incorrect? If it’s noise, you should drop or change them if you know the true values.
Does the outlier not change the results but affect the assumptions? In this case, you may drop the outlier or not.
Is the outlier affects both statistical results and the assumptions? In this case, we cannot merely drop the outlier. Try to run the analysis with or without the outlier and see the result.
Is the outlier create a significant association? If so, it is advisable to drop the outlier.
From a statistical standpoint, there is some suggestion for removing the outlier. If not, there is an option for transforming the data to pull in the high numbers or just using a different model.
The problem with these “outlier solutions” is that they also cause problems — biased parameter estimates and underweighted or valid values elimination.
What we need to remember; not all outliers are the same. Some have a strong influence, some not at all. Some are valid and important data values. Some are simply errors or Noise.
Truly understand your outlier, and your analysis might be better than before.
Here is a further read regarding Outlier you might want to know: